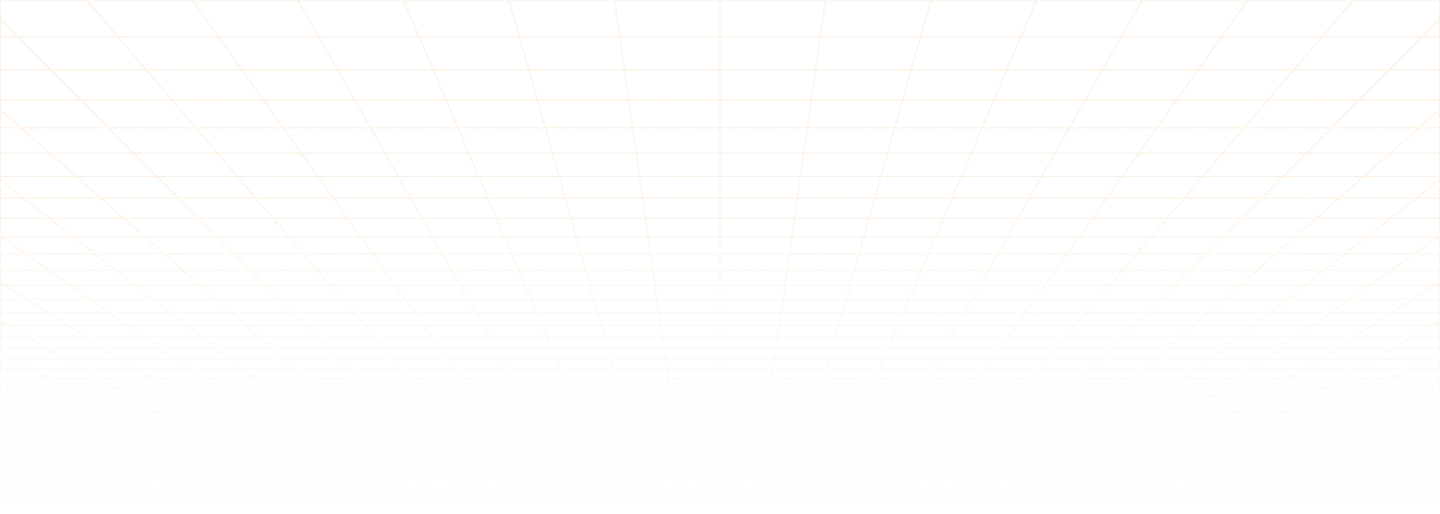
GPU scarcity is real, and the RTX 4090 is holding its own
Cloud computing is in a crunch. AI development is booming, but GPUs—especially top-tier ones—are harder to find than ever. Long waitlists, rising cloud costs, and overbooked clusters are slowing down teams who just want to fine-tune a model or run inference at scale.
In this context, developers are looking beyond traditional data center GPUs like the NVIDIA A100. High-performance consumer GPUs like the RTX 4090 are quietly gaining traction as a fast and affordable alternative. But how do they actually compare?
This article breaks down the trade-offs between the RTX 4090 and A100 for tasks like Retrieval-Augmented Generation (RAG) pipelines and running language models in the 7B–8B range. If you're figuring out what kind of compute makes sense for your next AI project—especially when every GPU-hour counts—this comparison is for you.
Architecture and compute performance
The NVIDIA A100, built on the Ampere architecture, has long been the go-to for large-scale training and inference. It comes with 6,912 CUDA cores and 432 third-gen Tensor Cores. On paper, it delivers around 19.5 TFLOPs of FP32 and 78 TFLOPs of FP16 compute.
The RTX 4090, a consumer card based on Ada Lovelace, offers 16,384 CUDA cores and 512 Tensor Cores. Thanks to its higher clock speeds, it reaches 82.6 TFLOPs in both FP32 and FP16—outpacing the A100 in raw throughput.
The A100 supports features like NVLink for high-bandwidth interconnects and Multi-Instance GPU (MIG) for partitioning. These are valuable in large-scale enterprise setups but add overhead for individual or bursty jobs. The 4090 doesn’t have these features—but it doesn’t need them for many common workloads.
Memory: how much do you really need?
The A100 has the edge on memory: 40 or 80 GB of HBM2e with up to 2.0 TB/s bandwidth. That’s ideal for training massive models or supporting wide context windows in RAG.
The RTX 4090 has 24 GB of GDDR6X with ~1.0 TB/s bandwidth. That’s plenty for running or fine-tuning models in the 7B–13B range, especially in FP16 or quantized formats. For most RAG tasks, 24 GB gives you enough headroom—unless you're pushing large batches or long prompts.
Benchmarks show the A100 40GB can process ~68 simultaneous prompts for a standard RAG task (1500 tokens in, 100 out). A 4090 will handle fewer, but still enough for typical development and small-scale production needs.
Training speed and precision trade-offs
For model training, both GPUs handle smaller LLMs well. The A100's large memory helps with batch size and model size flexibility. The 4090 can match it on throughput by using techniques like gradient checkpointing or lower precision formats like FP8 or int8.
In terms of raw speed, the 4090 holds its own. A ResNet-50 iteration completes in roughly the same time. For fine-tuning, experimenting, or pretraining smaller models, there's little reason to reach for an A100—especially when the cost gap is so wide.
The A100 wins on FP64 workloads and enterprise precision features like TF32, which matter in research or simulations, not most LLM use cases.
Inference and RAG throughput
Both GPUs are more than capable for inference. A 7B model like LLaMA-2 runs at around 120–140 tokens per second on either one. RAG tasks perform well on both—though the A100 handles higher concurrency better thanks to its memory.
In a typical RAG scenario, the A100 clocks in at ~2.3 seconds latency and ~2.8 requests per second. A well-provisioned 4090 setup can reach similar latency, especially with optimized memory management and batching.
The main difference shows up under pressure. If you're serving many users or large prompts, the A100 offers more headroom. If you're focused on cost and running smaller jobs, the 4090 hits a sweet spot.
Power-wise, the A100 is more efficient: 250–300W TDP compared to 450W for the 4090. But in cloud deployments, power efficiency only matters if it affects your bottom line. With pricing being what it is, the watt-per-token comparison usually favors the cheaper GPU.
Cost-performance in the real world
This is where the gap widens.
The RTX 4090 lists for about $1,599, while a used A100 can go for $10,000–$15,000—and that's for the 40GB model. In the cloud, A100 instances on major platforms hover around €3.40/hour. Services using 4090s can offer rates closer to €1.20/hour.
That’s a huge difference for nearly identical single-GPU performance in many tasks.
Some developers find that two RTX 4090s (costing under $4,000 total) can outperform a single A100 for less than a third of the price. That’s a big deal if you’re running fine-tuning jobs or hosting inference APIs without the backing of a hyperscaler.
Modern cloud providers are starting to offer multi-GPU 4090 instances—up to 8× per node. These setups deliver serious compute without the A100 price tag, often with high-spec CPUs, RAM, and fast SSDs bundled in. Some even offer 1 Gbps network and no data egress fees, making them ideal for training runs or spiky workloads.
Do GPUs affect output quality?
Not really. Evaluation frameworks like RAGAS measure your retrieval and generation quality, but those metrics don’t change based on your GPU. Whether you’re using an A100 or a 4090, what matters is your model, prompt engineering, and data quality.
If you're seeing poor RAG performance, the bottleneck likely isn’t your GPU—it’s how you’re using it.
Side-by-side: what’s the better choice?
Final thoughts
Both the RTX 4090 and A100 are excellent GPUs for AI workloads. But they’re built for different worlds.
The A100 is made for scaled-up training jobs, heavy inference loads, and enterprise-level infrastructure. It shines in clusters, not on a single developer’s desk.
The RTX 4090, meanwhile, delivers incredible performance for its price. It’s perfect for developers running 7B models, building RAG pipelines, or experimenting with fine-tuning. And when GPU scarcity makes A100s hard to come by—or prohibitively expensive—instances based on the 4090 are often the practical choice.
Some platforms now offer up to 8× RTX 4090s in a single node. That kind of firepower, paired with transparent pricing and fast provisioning, unlocks a lot of possibilities for teams that need power without the enterprise baggage.
In the end, it’s not about which GPU is “better.” It’s about what’s available, what you’re building, and how much you're willing to spend. And right now, the RTX 4090 ticks a lot of the right boxes.